How Much Should We Trust Empirical Estimates of State Corporate Law Effects?
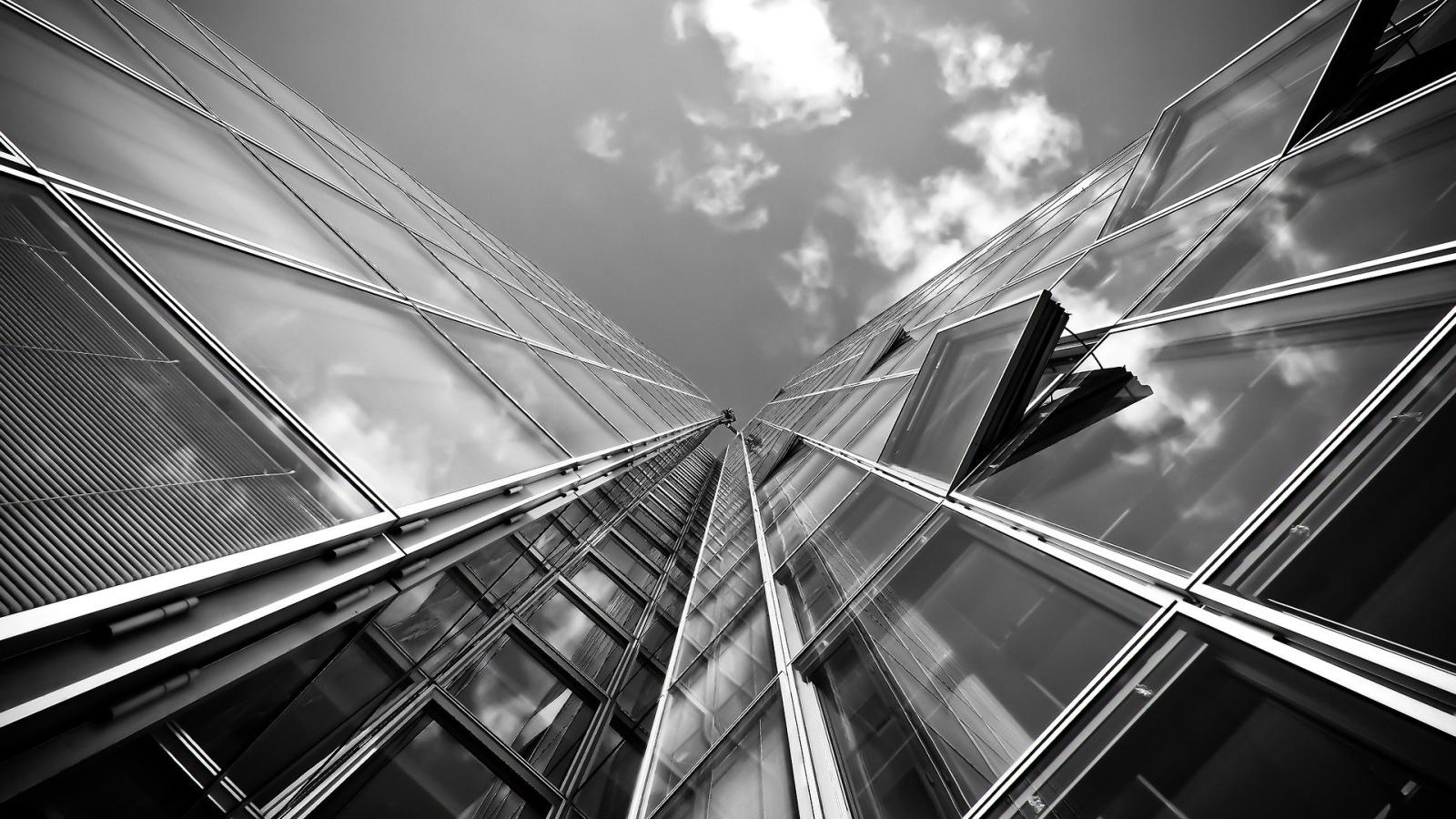
A large empirical literature studies the effects of U.S. states’ corporate law statutes on corporate actions and performance at the firm level. Interest is in the laws for their own sake or as exogenous variation in determinants of firm behavior. For example, well over a hundred papers investigate the effects of state anti-takeover statutes or of state universal demand laws. How much should we trust the estimates of these papers? The short answer is: not much. A brief non-technical explanation follows. The full technical explanation is available here. (Quick technical summary: the conventional clustered standard errors are much too small due to the extreme cluster size imbalance.)
Like any empirical study, studies of state corporate laws have to address two potential problems: bias and noise. Bias refers to a systematic deviation of the effect estimate from the true causal effect. For example, a particular type of state might be more likely to adopt a particular type of statute and attract a particular type of firm, such that any statistical association between statute and firm outcome might be driven by selection of firms into states rather than any causal effect of the statute. Avoiding this potential bias is one reason why the vast majority of empirical studies estimate changes in firm outcomes as a function of staggered changes in state laws in a so-called difference-in-difference design. This design holds fixed individual firm’s average outcomes with so-called firm fixed effects, and usually controls for year-to-year changes common to all firms or to all firms in an industry etc. with year fixed effects or year-industry fixed effects etc. (other controls are recommended as well). Another reason to control for firm and year effects is to absorb the noise that results from firm-to-firm and year-to-year variation. Noise is random variation that is not systematically related to the variables of interest but that can obscure their relationship. The less noise, the easier the detection of true effects.
Still, noise will inevitably remain even with controls. The question is how to tell evidence of a true effect from the remaining noise. This is known as the problem of statistical inference. The basic approach of classical hypothesis testing starts by estimating from the data not only the effect under consideration—the so-called point estimate—but also the noise. One rejects the null hypothesis only if the point estimate is sufficiently large relative to the noise estimate, i.e., if a point estimate of this size seems sufficiently unlikely to arise by mere chance, given one’s noise estimate. In social science, “sufficiently unlikely”—the so-called “size” of the test—is usually chosen to be 5% or 10%.
In complex settings such as the study of state laws, estimating the noise is not straightforward. One major complication is that the noise in individual firm-year observations is not independent from one another. Noise is likely to be correlated not only within each firm from one year to the next, but also within each state both within one year (i.e., across firms in that state) and from one year to the next. The reason is that states can take various actions that will affect all firms incorporated there for many years, and not all of these actions can be explicitly accounted for by the statistical analyst. The consequence is that one has far fewer observations to estimate the noise than would at first appear – in essence, one has only as many observations as there are states of incorporation in the sample. To deal with this issue, it has become standard practice to “cluster” the noise estimates by state of incorporation. This technique can yield valid estimates of the noise even in the presence of arbitrary within-cluster dependence. The technical argument for the validity of the noise estimate is asymptotic—i.e., it holds only as the number of clusters tends to infinity. Standard advice in the general methodological literature is that 50 clusters are sufficient in practice, which would mean the technique should work with state corporate laws. However, the standard advice usually comes with the ominous caveat that more clusters might be required if the cluster sizes are unbalanced, i.e., if the number of individual observations differs between clusters. In the state corporate law context, cluster sizes are extremely unbalanced, chiefly but not only because Delaware is home to more than half of all public corporations. This suggests there could be a problem with the usual noise estimates in empirical studies of corporate law. In my paper, I show that there is indeed a problem—a big problem!
My main way of demonstrating the problem is with Placebo laws. That is, I randomly draw states and years and then run a usual study design as if laws had been enacted in those states and years. In fact, I do that tens of thousands of times to study the distribution of estimates that result from such random draws. Since the draws are random, they have nothing to do with any real law. By mere chance, individual draws could still be correlated with firm outcomes. However, if the statistical inference described above worked, it should be able to tell that these correlations are just noise, up to the specified type I error rate a/k/a test size. That is, one should occasionally falsely infer that a Placebo law causes firm outcomes, but this should not happen more often than the specified test size. Unfortunately, it happens much more often. The exact numbers depend on some details such as the number of states that are randomly drawn as “treated” by the (inexistent) law. On average, the conventional test falsely finds an effect 9/21/30% of the time if the nominal test size is 1/5/10%. The upshot is that results in the empirical literature on corporate laws are much more likely to be mere noise than suggested by their reported “p-values” (i.e., probability of a type I error).
In the rest of the paper, I explore the source of the problem and possible fixes. The source of the problem is the very unequal cluster sizes, i.e., number of firm-years per state. Delaware’s concentration of more than half of all publicly traded firms is the main culprit, but it is not the only one: Even without Delaware, average rejection rates are 6.4/13.9/20.4% for nominal 1/5/10% tests. The fixes commonly recommended in the methodological literature fail in the corporate law setting, including the cluster wild bootstrap. I propose a permutation test that is exact under some assumptions, and in simulations performs better than the alternatives even when the assumptions do not hold.