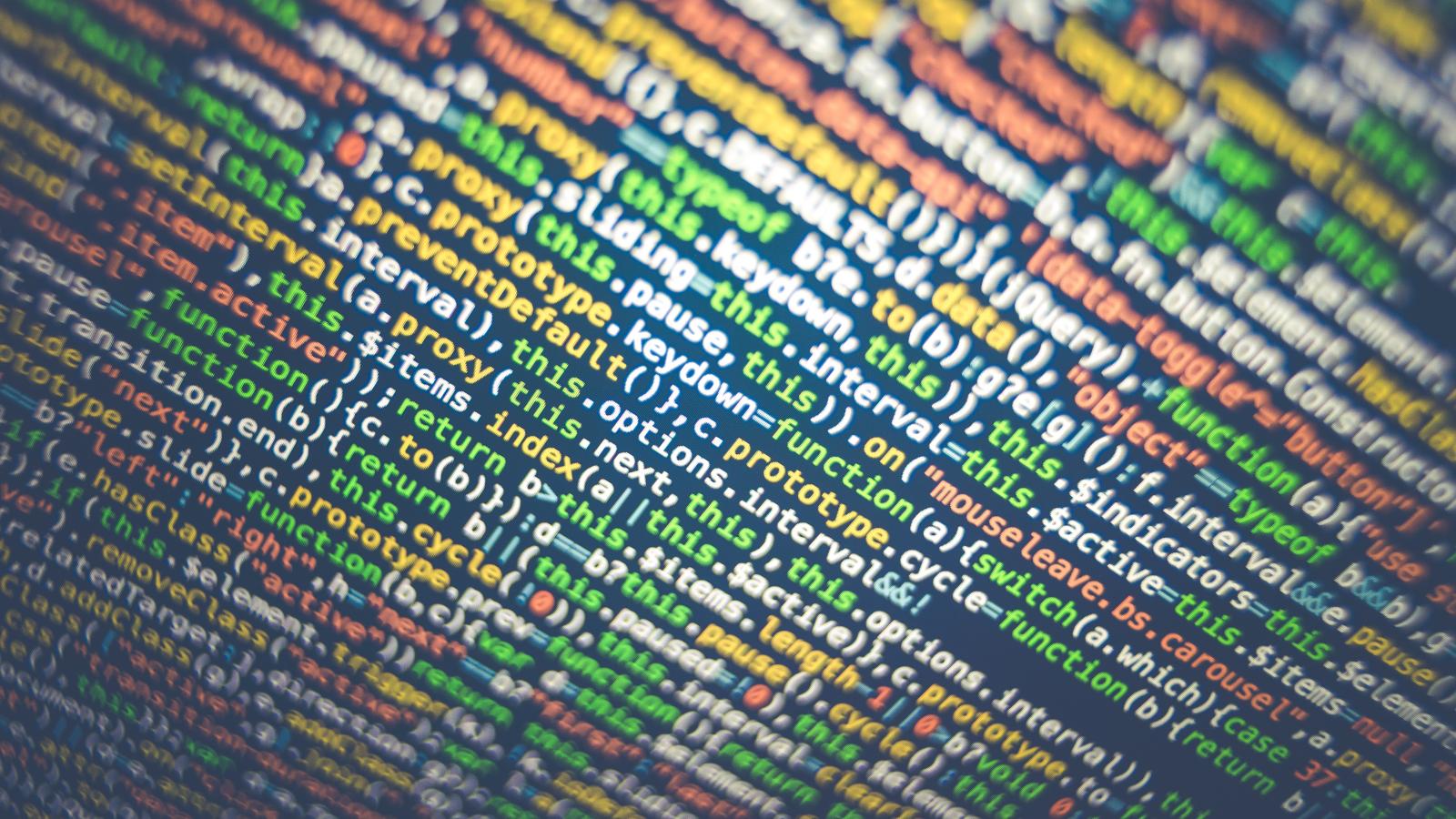
The ECGI blog is kindly supported by
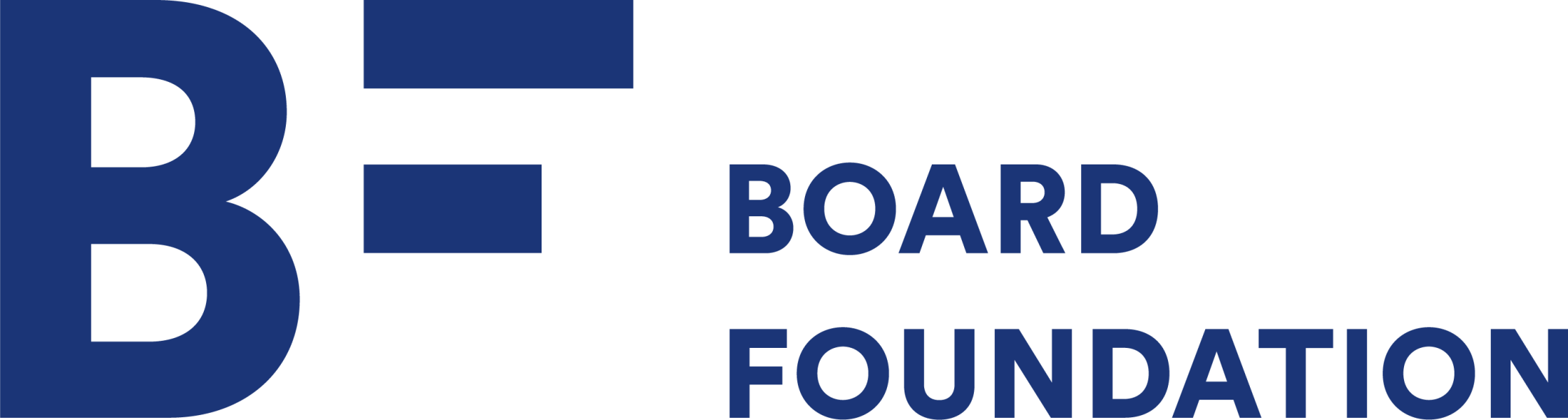
Economic and Normative Implications of Algorithmic Credit Scoring
Commercial use of artificial intelligence (AI) is accelerating and transforming nearly every economic, social and political domain. Companies have been attempting to classify and label items, processes, and people for a long time. The modern convergence of foundational technologies, however, enables the analysis of vast amounts of data required for AI. Consider how much data is created and used based on our online behaviours and choices. Converging foundational technologies enables analytics of the vast data required for machine learning. As a result, businesses now use algorithmic technologies to inform their processes, pricing and decisions.
Lending has been identified as a high risk for discriminatory algorithms where using historical data that will result in biased algorithmic tools. Bias, among other risks, is an essential consideration. However, there is a gap in recent literature on the potential optimal outcomes that can arise if risks are mitigated. Algorithmic credit scoring can significantly improve banks’ assessment of consumers and credit risk, especially for previously marginalised consumers. It is, therefore, helpful to examine the commercial considerations often discussed in isolation from potential normative risks.
We should not so readily dismiss the potential benefits of well-designed tools.
In a recent paper “Algorithmic decision-making in financial services: Economic and normative outcomes in consumer credit” (AI and Ethics), I aim to challenge the persistent assumption that the use of algorithmic credit scoring and alternative data will only result in discriminatory outcomes or harm consumers. We should not so readily dismiss the potential benefits of well-designed tools. Initially studied in isolation, ethical concerns will benefit from intersectional research alongside corporate perspectives.
Consider the notable example where the Apple Card (underwritten by Goldman Sachs Bank USA) was widely criticised for alleged discrimination against female credit card applicants, especially on social media. Some women were offered lower credit limits or denied a card, while their husbands did not face the same challenges. The claims sparked a vigorous public conversation about the effects of sex-based bias on lending, and the hazards of using algorithms and machine learning to set credit terms. The New York State Department of Finance investigated the algorithms involved and concluded there were valid reasons for these instances of disparity and could not find any discriminatory practices. The Department acknowledged that there are risks in algorithmic lending, including "inaccuracy in assessing creditworthiness, discriminatory outcomes, and limited transparency".
First, I examine the economic implications of using machine learning to address traditional challenges in consumer credit contracts. These include information and power asymmetry between banks and consumers, as well as conflicting interests and incentives. Then, I consider the critical aspects of machine learning that dispel some misconceptions about algorithmic credit scoring. I explain how banks use machine learning to classify people and calculate credit scores and how they can use it to predict future consumer behaviour. Finally, the article evaluates risks that, if mitigated, could potentially improve economic and normative outcomes in the traditional consumer credit contract market.
These economic and normative issues include:
- Whether ML increases the accuracy of the creditworthiness assessment of consumers;
- The potential for ML to make more efficient pricing structures and provide a competitive advantage for banks with more accurate models;
- If introducing algorithmic decision-making to the financial sector can further erode consumer trust and institutions’ reputations;
- The incongruity between improving accuracy and protecting consumers’ privacy and autonomy;
- The risk of ML replicating or compounding injustice and resulting in discriminatory algorithms.
There is considerable concern about the risk of algorithmic bias and discrimination in the context of credit institutions using ML. I highlight biases towards specific personal characteristics, such as race, gender, marital status or sexual orientation, that have historically impacted loan and credit decision-making processes. ML in credit scoring and access to financial services has amplified these concerns. Then, I consider the various technical fairness metrics proposed to overcome algorithmic bias and note that each metric requires different assumptions. This tension is exacerbated by the trade-off between fairness and accuracy when ML models are designed to prefer a certain level of fairness.
Normative questions about the moral framework that guides AI cannot be divorced from questions about how we evaluate the moral framework that guides corporations.
Such trade-offs are challenging for financial institutions, which like most companies, will continue to function with the prioritisation of profit. However, the future of corporations may shift with the knowledge, as described by Larry Fink, that "in fact, profits and purpose are inextricably linked". At the same time, as many consider the purpose and values of corporations, there is a similar impetus for the ethical design of AI.
Normative questions about the moral framework that guides AI cannot be divorced from questions about how we evaluate the moral framework that guides corporations. The reason is that, despite the misnomer, this view treats AI as ephemeral or autonomous, not as tangible decision rules and utility functions of the architect.
My article makes two essential contributions to the literature on the corporate use of algorithmic decision-making. First, examining the outcomes of using ML from a combined economic and normative approach is unique and allows for more rigorous consideration of the real-world costs and benefits. Second, despite the risk of harm that many experts in the field have identified, there is a clear opportunity to design ML. This will improve and optimise economic and normative outcomes. I propose a renewed enthusiasm for the potential positive outcomes.
I conclude that future work on regulatory issues should consider the underlying incentives and interests that shape behaviour in this area.
------------------------------------------------------------------------------------------------------------
By Holli Sargeant, a PhD Candidate in the Faculty of Law, University of Cambridge.
For further reading on this topic, read 'Regulating for “humans-in-the-loop”' by Talia Gillis.
The ECGI does not, consistent with its constitutional purpose, have a view or opinion. If you wish to respond to this article, you can submit a blog article or 'letter to the editor' by clicking here.
This article was originally published on the Oxford Business Law Blog