
The ECGI blog is kindly supported by
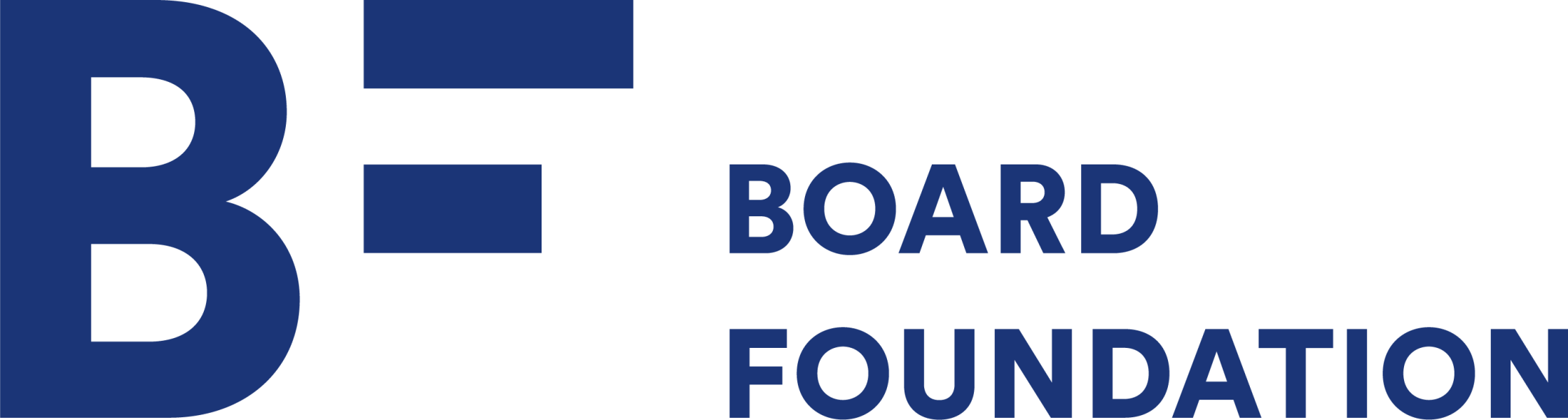
Artificial Intelligence and the “S” in ESG
Googling “ESG” gives you half a billion results. It has become a generic term, used by politicians, regulators, research scholars and corporates, lumping together pretty diverse goals for how to run a company. While the “G” has a long pedigree in corporate governance research and business practice, this is not the case for the other two. Lawmakers and financial regulators around the world have been busy defining novel taxonomies for the “E”. The “S” currently seems to encompass all things “social”, ranging from diversity on boards to human rights in supply chains.
One of the less controversial ingredients of “S” is a commitment to anti-discrimination efforts. In the US, racial equity audits are suggested as a part of ESG. In the EU, non-discrimination figures prominently in early stage plans to establish a social taxonomy. At the same time, many doubt that shareholder value is compatible with private companies actively engaging in anti-discrimination, understood as doing more than what the law requires anyway.
Grappling with the tension between a company’s business case and anti-discrimination efforts, artificial intelligence (AI) has been understood as promising.
Grappling with the tension between a company’s business case and anti-discrimination efforts, artificial intelligence (AI) has been understood as promising. AI credit scoring provides an example for how this might work for financial institutions. The decision to hand out and price credit entails an assessment of the borrower’s credit default risk. Faced with conditions of uncertainty, transaction costs and imperfect competition, lenders depend on access to (hidden) fundamental information about borrowers. Credit scoring agencies support lenders by relying on a limited number of variables which enter in a score to guide the credit decision. However, depending on the variables chosen, “thin-file” minority applicants will not always see their (low) score adequately reflect their real credit default risk. Hence, including minority borrowers becomes a question of search costs, balanced against the expected return on a loan to the applicant. In the past, few lenders have found it cost-efficient to invest in finding “invisible prime” candidates.
Cheap access to big data and ease of AI modelling via machine learning might change that equation. For minority borrowers, inclusion through AI seems possible, especially if compared with either the limited list of input variables of traditional scoring bureaus or the biases and cognitive limitations of human credit officers. A good record as to ESG-compliance might be an attractive add-on from the lender’s perspective.
Because AI is trained on past data which include the traditional variables as well as decisions taken by human credit officers, the AI develops its own biases
Unfortunately, things are rarely as straightforward as the search cost argument suggests. Two reasons for that standout. The first has to do with biases. Because AI is trained on past data which include the traditional variables as well as decisions taken by human credit officers, the AI develops its own biases, often deepening existing ones. There is usually no counterfactual data on loans which would have been attractive for the lender but were not granted by the loan officer. Hence, the AI cannot learn from mistakes in such decisions. This compounds the problem as does over-reliance on AI. Even if a lender employs “human in the loop” procedures, the loan officer will often defer to what the AI suggests, doubting that his assessment beats the computational power of the machine.
Technology produces winners and losers. Where you find yourself depends on the correlations the AI singles out
The second reason has to do with statistical discrimination. (Theoretically) assuming competitive markets, risk-neutral lenders, and interest rates contingent on borrower characteristics, we would expect differences in access to loans and in interest rates to be signs of (necessary) statistical (not taste-based) discrimination. But empirical studies point in a different direction, showing that technology produces winners and losers. Where you find yourself depends on the correlations the AI singles out to produce an attractive risk-reward case for the lender. If you are vulnerable to strategic pricing, as (in the US) Blacks and non-white Hispanics often are, you might end up among the losers. Put differently: AI will further inclusion only for some. Taken together with the biased AI problem, these might not be the ones you were looking for.
What is the take-away from this example? Credit scoring illustrates great potential for ESG’s “S”, with the AI lowering search costs. But lenders must carefully distinguish decision-supporting from decision-making. Responsibility for the latter rests with humans, not machines. And this might be true for the use of AI in most, if not all corporate decisions.
Dr. Katja Langenbucher is Professor for civil law, commercial law and banking law at Goethe-University's House of Finance and ECGI Research Member.